Genomics: Insight
Pneumonia biology through the lens of machine learning and AI: current trends and challenges
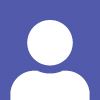
Hypothesis: Combining digital pathology with spatial transcriptomics/genomics can unveil molecular level insights & help elucidate the host response to pneumonia.
Introduction
Pneumonia is characterized by the inflammation in the lungs from a persistent infection leading to disruption of gas exchange in the lower respiratory tract. Though the onset of pneumonia is acute, and primarily affects the lungs, it may have extrapulmonary effects which can result in prolonged morbidities2. Elderly patients (>70 years), especially the ones with existing co-morbidities, and young children (under 5 years) are the two demographics that are severely affected by pneumonia3. Severe forms of pneumonia can lead to Sepsis/Acute respiratory distress syndrome (ARDS); however, the nature and the extent of the host response largely determines the outcome2. Hence, it remains crucial for us to study and understand the host response to pneumonia.
Pneumonia remains the first leading cause of death among infectious diseases1 and eighth among all diseases with a significant disease burden. Pneumonia can be caused by many different pathogens, including bacteria (e.g. Streptococcus pneumoniae, Klebsiella pneumoniae), viruses (e.g. influenza A virus, coronaviruses) and fungi (e.g. Aspergillus fumigatus, Cryptococcus neoformans). However, the causal agent cannot be identified in a majority of pneumonia patients due to use of antibiotic treatments that leads to pathogen clearance, difficulties linked to collecting specimens from lower respiratory tract etc.,2. Even when the pathogen is detected, patients exhibit heterogenous and non-specific responses making the diagnoses, treatment and understanding of pneumonia extremely complex. Hence it could benefit to focus further on the host-response to pneumonia.
Artificial Intelligence (AI) is a field of computer science that refers to developing computational models with human-like reasoning capacity. Machine learning (ML), a sub-field of AI that aims to build models that are trained on large datasets and are capable of making “decisions” on the new and previously unseen cases based on the knowledge gained from the training data. Deep Learning (DL) is a field of machine learning that uses a more complex set of operations such as neural networks, to build models that can do tasks similar to machine learning. Digital pathology analysis leverages machine learning to automatically detect abnormal features in disease tissue. Spatial transcriptomics/genomics methods can capture gene expression changes & spatial distribution in tissue sections that is crucial to disease biology7.
Recent advances in machine learning and AI have expanded our ability to apply decision-making capabilities to other fields including health care and medical research4-6. ML algorithms have begun to help us understand pneumonia by mapping the location with gene expression patterns specific to a particular cell type using spatial omics & by parsing imaging data to identify patterns of host response. Combining digital pathology with spatial transcriptomics to obtain molecular level insights holds promise for elucidating the host response to pneumonia.
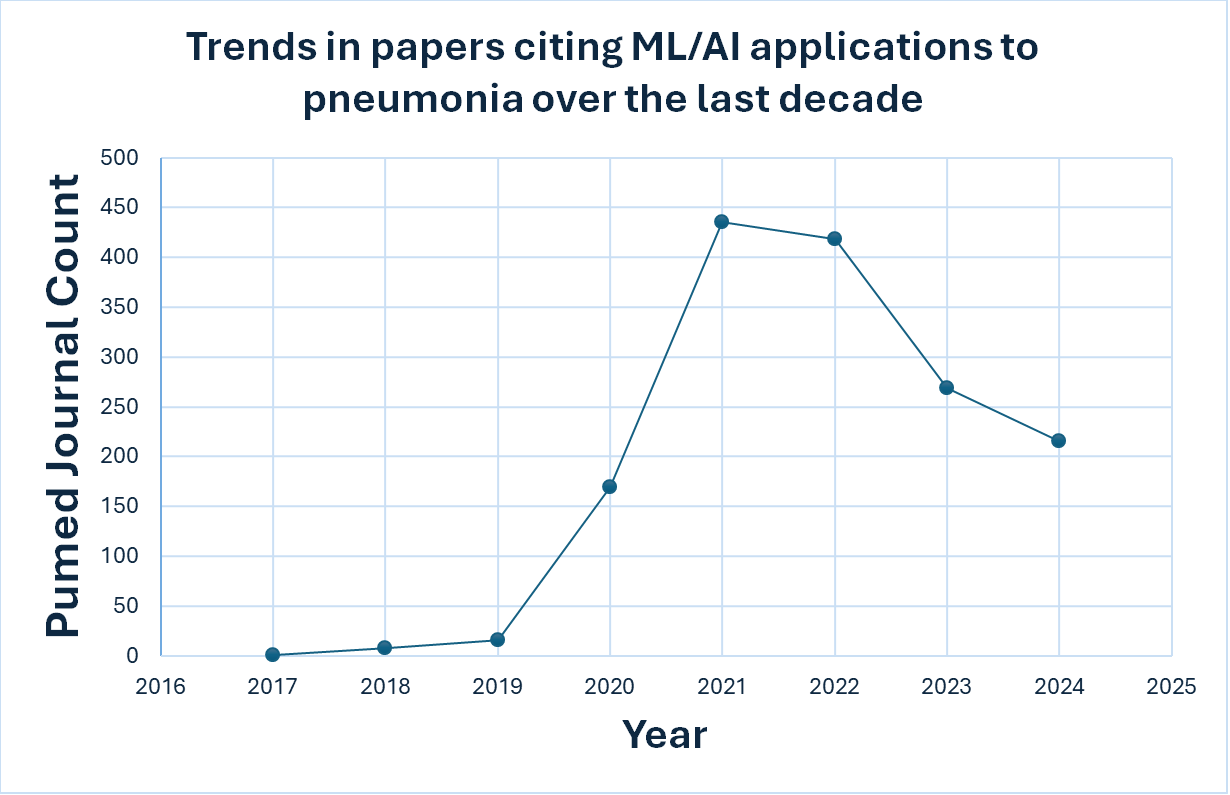
Figure 1. Recent trends in ML application to Pneumonia. This figure was generated using count data obtained from querying the PubMed with the search terms pneumonia, machine learning, artificial intelligence, and deep learning.
Current trends in the application of ML/AI models to pneumonia biology
Application of AI/ML methods to pneumonia biology can be broadly classified into 2 groups. Firstly, we have the image-based application that explores chest CT (computed tomography) and X-ray (radiograph) images to detect/diagnose pneumonia in patients8-12. Next, we have methods that are modeled on clinical data & mined text for mortality prediction & treatment and genomics data for the characterization of cellular and molecular nature of the disease.13-15. Both methods have made remarkable strides in their respective applications, however, image analysis remains the popular option due to limited availability of clinical datasets. We have seen a tremendous growth in studies that have applied ML/AI models to pneumonia. Recently published studies from PubMed containing key words “Pneumonia”, “Machine learning”, “Artificial Intelligence“ and “Deep learning” have increased greatly since 2020 (Figure 1). We can see an uptick in papers around 2020/2021 as the COVID-19 pandemic increased the need for quick diagnostic approaches as severe forms of COVID-19 leads to pneumonia. In addition to the pandemic, ML/AI achieved considerable advances in terms of performance and successful applications in other fields, which boosted their interest in application to healthcare problems.
Diagnosis of pneumonia is complex and can result in misdiagnosis because diagnosis is often made based on non-specific physical symptoms and imaging data that are open to varied interpretation16. Recent evaluation of DL models on radiographs (X-ray) showed that DL models sometimes outperformed radiologists, reduced diagnostic time and resolved confusion/misinterpretations with a suspicious chest radiography17.
Challenges and future directions
Explainability of pneumonia biology
DL prediction models learn from the features (textures, lines, edges etc.,) available in the images based on the labels. Models can predict things such as whether a person has pneumonia and whether the clinical features show patterns of earlier mortality. However, these models fail to explain the “why” behind its decision18. Although recently implemented explainability methods such as feature importance which identifies features that are driving the prediction or class activation maps which highlights the part of image influencing the prediction such as tumor in an image are promising, there are issues with integration, robustness and specificity of results which hinder proper implementation of these methods alongside training models in the medical field19,20. Further, using only the CT/CR images to understand the disease biology adds to the complexity.
Limitation of classification and prediction
Current image analysis methods are mostly focused on diagnosis or prognosis. These tools provide little insights to understand the disease biology including different aspects of patient response that can play a crucial role in response to treatment. Machine learning/AI can be leveraged for methods beyond this simple classification – to explore the underlying cellular, molecular and spatial biology using spatial transcriptomics and digital pathology.
The role of digital pathology & genomics in pneumonia
In pneumonia, we see heterogeneous host response even within the same etiology and a comprehensive representation of the host-response heterogeneity is critical. Imaging data such as CT/CR fails to capture the underlying cellular and molecular changes which plays an important role in defining the host response. One way to capture the changes at a cellular level is to look at sections of diseased tissue using histopathology. Pathological assessment of tissue can provide a comprehensive overview of the underlying immune and cellular environment. Measuring histopathology features such as necrosis can inform the extent of cell death in the tissue and understanding this could guide the development of specific treatment plans to combat the increase in cell death. Further, the ability to automatically quantify several other features such as hemorrhage, metaplasia and neutrophil levels using digital pathology can help us understand the range and severity of pneumonia very quickly.
Histopathology, however, fails to capture the molecular changes that is critical to understanding the host response in pneumonia. One way to overcome this would be to use spatial transcriptomics/genomics technologies in combination with digital pathology to gain insights into pneumonia.
Analyzing histopathology images remains a daunting task as it takes hours for a pathologist to review the tissue morphology and determine the severity of disease features. Even then, there is very less consensus amongst pathologists diagnoses on the same data. There is a need to develop methods that can rapidly examine the complex tissue heterogeneity and capture the levels of individual histopathology features such as fibrosis and necrosis. By quantifying real biological features, these models will be interpretable to pathologists and clinicians further increasing their utility.
Despite the promise of digital pathology, collecting large numbers of tissue samples is challenging as lung biopsies are not obtained as standard of care in this setting. Another potential avenue for the collection of pneumonia tissue samples is post-mortem lungs collected at autopsy. However, the number of centers that can perform rapid autopsies with appropriate tissue processing is relatively small. Furthermore, labeling these tissue sections with expert annotations is a challenging task. Due to the varied interpretation of features amongst pathologists, it is important to have more than one pathologist to create labels with stronger consensus.
If the challenges of tissue collection, dataset generation, and expert annotations can be overcome, building of machine learning models that can automate the detection of disease features and provide insights into the host response quickly and efficiently may become a reality.
It remains a priority to build machine learning models that can automate the detection of tissue heterogeneity.
Conclusions
Host response to viral or bacterial pneumonia is heterogeneous. To better understand these differences, there is a need to build specific AI/ML methods that can rapidly dissect different disease phenotypes. Existing applications of AI/ML methods are focused on the
classification/prediction tasks and lack the ability to look beyond predicting mortality or diagnosis. Future applications need to consider the complexity of pneumonia at the tissue level. We propose that it could be beneficial to look at different kinds of images such as histopathology supplemented by spatial transcriptomics which can provide a more detailed overview of host response. Though obtaining histopathology sections is invasive, even exploring a small subset of existing sections could help build models that could trace the cause behind features such as high cell death or fibrosis in pneumonia. Potentially, these models can act as a guiding system to speed up the process, help inform care decisions, process, or resolve conflicting evidence.
References
- Jain, S. et al. Community-Acquired Pneumonia Requiring Hospitalization among U.S. Adults. N Engl J Med 373, 415–427 (2015).
- Quinton, L. J., Walkey, A. J. & Mizgerd, J. P. Integrative Physiology of Pneumonia. Physiol Rev 98, 1417–1464 (2018).
- Torres, A. et al. Pneumonia. Nature Reviews Disease Primers 2021 7:1 7, 1–28 (2021).
- Sidey-Gibbons, J. A. M. & Sidey-Gibbons, C. J. Machine learning in medicine: a practical introduction. BMC Med Res Methodol (2019).
- Oh, S. H., Lee, S. J. & Park, J. Precision Medicine for Hypertension Patients with Type 2 Diabetes via Reinforcement Learning. J Pers Med (2022).
- Qiu, W. et al. Interpretable machine learning prediction of all-cause mortality. Communications Medicine (2022).
- Wang, W. J. et al. Spatial transcriptomics: recent developments and insights in respiratory research. Military Medical Research Preprint (2023).
- Hemdan, E. E.-D., Shouman, M. A. & Karar, M. E. COVIDX-Net: A Framework of Deep Learning Classifiers to Diagnose COVID-19 in X-Ray Images. (2020).
- Moradi Khaniabadi, P. et al. Two-step machine learning to diagnose and predict involvement of lungs in COVID-19 and pneumonia using CT radiomics. Comput Biol Med (2022).
- Lee, S. M. et al. Deep Learning Applications in Chest Radiography and Computed Tomography. Journal of Thoracic Imaging Preprint (2019).
- Yang, Z. Y. & Zhao, Q. A multiple deep learner approach for X-Ray image-based pneumonia detection. in Proceedings - International Conference on Machine Learning and Cybernetics (2020).
- Kareem, A., Liu, H. & Sant, P. Review on Pneumonia Image Detection: A Machine Learning Approach. Human-Centric Intelligent Systems (2022).
- Elkin, P. L. et al. NLP-based identification of pneumonia cases from free-text radiological reports. AMIA ... Annual Symposium proceedings / AMIA Symposium. AMIA Symposium (2008).
- Chang, T. H. et al. Clinical characteristics of hospitalized children with community-acquired pneumonia and respiratory infections: Using machine learning approaches to support pathogen prediction at admission. Journal of Microbiology, Immunology and Infection (2023).
- Effah, C. Y. et al. Machine learning-assisted prediction of pneumonia based on non-invasive measures. Front Public Health (2022).
- Ginsburg, A. S. & McCollum, E. D. Artificial intelligence and pneumonia: a rapidly evolving frontier. The Lancet Global Health Preprint (2023).
- Becker, J. et al. Artificial Intelligence-Based Detection of Pneumonia in Chest Radiographs. Diagnostics 12, 1465 (2022).
- Hamilton, A. J. et al. Machine learning and artificial intelligence: applications in healthcare epidemiology. Antimicrobial Stewardship & Healthcare Epidemiology 1, e28 (2021).
- Meyes, R., de Puiseau, C. W., Posada-Moreno, A. & Meisen, T. Under the Hood of Neural Networks: Characterizing Learned Representations by Functional Neuron Populations and Network Ablations. (2020).
- Chaddad, A., Peng, J., Xu, J. & Bouridane, A. Survey of Explainable AI Techniques in Healthcare. Sensors Preprint (2023).
About the Author
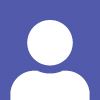
Ms. Amulya Shastry is a PhD Candidate at the laboratory of Dr. Joshua Campbell, Dr. Stefano Monti, and Dr. Joseph P. Mizgerd. Her work focuses on applying machine learning methods to decipher pneumonia biology. Dr. Bradley Hiller is a postdoctoral research fellow in the laboratory of Dr. Joseph Mizgerd at the Boston University Pulmonary Center. His work focuses on developing and characterizing mouse models which reflect human lung disease.
Academic Mentors: Drs. Joshua C, Stefano M, Joseph PM Affiliation: Boston University