Genomics: Insight
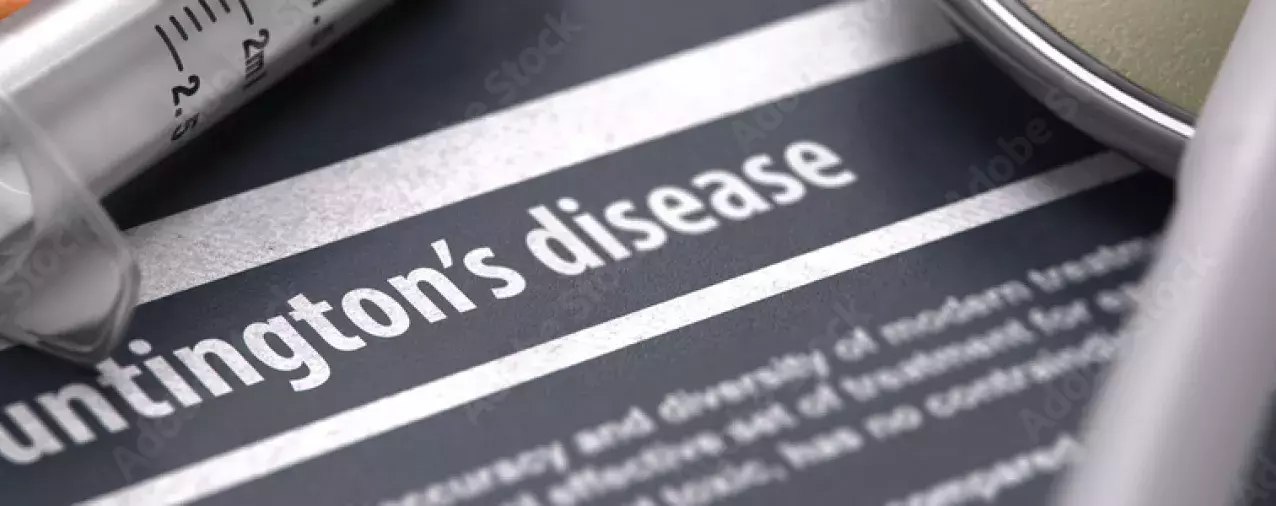
Examining Correlations Between Environmental Variables and Huntington’s Disease Symptoms
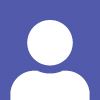
Hypothesis: Environmental variables will correlate with earlier onset of symptoms for Huntington’s Disease.
Background
Huntington’s disease (HD) is an autosomal dominant (genetically inherited) neurodegenerative disorder whose worldwide prevalence is equivalent to 2.71 per 100,000 people. This incidence rate notably rises to 5.70 in North America, Western Europe, and Australia. The increased frequency of HD among Western populations has been attributed to a higher occurence of the huntingtin (HTT) gene in western haplotypes (12-marker Y chromosome associated with haplotype R1b1a2, the most common haplogroup in Europe).1 HD is caused by the polyglutamine expansion mutation, in which DNA segments repeat the sequence CAG multiple times in a row in the HTTgene.2 This mutation generates the defective protein huntingtin (mHTT).3 HD results from mutations of the HTTgene located on the short arm (p) of chromosome 4 (locus 4p16.3).4 Disease prevalence depends on average polyglutamine length. The longer the CAG repeat length, the more likely HD early onset (when symptoms of the disease first become detectable) will occur. HD causes dysfunction in numerous areas, mainly proteostasis, transcription, axonal transport, synaptic activity, and neurotransmitter signaling. The condition’s symptoms can manifest in three areas: motor, cognitive, and neuropsychiatric. Motor symptoms include chorea (early stage), bradykinesia, dystonia, and rigidity (later stages); cognitive symptoms include issues with memory, attention, and executive function; neuropsychiatric symptoms can include depression, anxiety, and irritability. Current therapeutic options for HD include chorea medications, which inhibit dopamine receptors, antidepressants, antipsychotic medication, and mood stabilizers.5 Although widely regarded as a disease determined solely by genetics, HD symptoms have demonstrated correlations to environmental variables, particularly lifestyle factors, socioeconomic status, and mental health.
Environmental Variables
After a review of recent studies, Lund et al. report positive associations between poverty indicators, such as educational levels and socioeconomic status, and common mental health disorders. Cubo et al. and Callouri et al. found positive associations between lifestyle factors and the onset and progression of HD symptoms. We will examine three environmental factors that demonstrate connections to CAG repeat variation.6
Lifestyle
CAG repeat length correlates with lifestyle factors, including environmental exposure to genotoxic agents, alcohol, and tobacco, as well as dietary factors. . Jonson et al. found a correlation between exposure to genotoxic agents such as hydrogen peroxide, potassium bromate, and methyl methanesulfonate known to induce DNA damage and CAG repeat length increases.7 Cubo et. al found that individuals with a higher BMI than the low BMI common in HD patients (with a hazard ratio of 86%), individuals with mild alcohol consumption, as opposed to heavy alcohol consumption (with a hazard ratio of 12%), and individuals with consuming caffeine (with a hazard ratio of 13%) slowed the progress of HD symptoms. Moreover, active lifestyles (including active cognitive lifestyles) may delay the onset of HD symptoms.8 As Mo et al.’s review covers, in mouse models of HD, increased cognitive and sensorimotor stimulation can delay the onset HD symptoms.9 As for dietary factors, Berggren et al. found that neonatal iron supplementation has associations with oxidative stress that exacerbates HD symptoms.10 Conversely, a polymeric nutritional supplement that enriches caloric intake can improve HD patients’ BMI to healthy levels, which shows that changes in diet can combat symptoms. Changes in diet can also downregulate several microRNAs that are upregulated in HD. Additional interventions include introducing omega-3 polyunsaturated fatty acids to patients’ diets, which can improve the quality of life and slow the progression of symptoms, including cachexia and weight loss in HD patients. Adherence to a Mediterranean diet may also correlate with slowing the progression of symptoms in HD patients, with patients consuming Mediterranean diets being 14% less at risk for premature death.8
Socioeconomic Factors
Following an analysis of 67 million insurance enrollees in the United States from 2003-2016, where 3,707 individuals were diagnosed with HD, Bruzelius et al. identified several previously unreported differences in HD frequency by factors such as self-reported gender and income. The study found that diagnostic frequency, referring to the onset of symptoms, decreased from 8.52 per every 100,000 persons to 4.79 when annual household income increased from less than $49,000 to $100,000 or more. The authors identified a similar trend when examining age-adjusted diagnostic frequency to the 2000 U.S. population. The study identified a parallel trend with educational attainment levels, where diagnostic frequency rates decreased as attainment increased. The authors identified possible reasons for socioeconomic impacts on HD, including that individuals experienced fiscal losses after cognitive symptoms manifest.11 Mendizabal et al., in a study of ENROLL-HD respondents, found that participants with less than a high school degree demonstrated lower Total Functional Capacity Scores (TFCs), a marker of disability due to HD, than patients with higher education or professional degrees. The authors determined a correlation between lower educational levels and greater severity of disability from HD symptoms.12 Murillas et al. furthermore substantiate the association between educational attainment and HD symptom progression; the authors found a significant correlation between lower years of education, fewer correct responses in a verbal fluency task to evaluate HD symptoms, and higher social vulnerability index score.13 Another study done by Wexler et al. noted that as much as 60% of variation in CAG expansion correlates to environmental factors, including socioeconomic class. The authors analyzed HD prevalence in Venezuelan kindreds, finding among sibling pairs that 84% of the variance in the age of onset was not attributed to the HTT gene itself but to other environmental factors, including correlations to outside pollutants, marginal diets, and extreme poverty levels shared across the sample selection.14
"as much as 60% of variation in CAG expansion may be due to environmental factors"
Mental Health Factors
A study done with salivary cortisol and Inventory of Depressive Symptomatology (Self Report) among pre-HD (preceding detectable HD symptoms) and early-onset HD patients (symptoms detectable) found that patients depressed prior to showing symptoms for Huntington’s Disease (pre-HD), had higher cortisol levels when they were early-onset HD. Early-onset HD patients were shown to have an increased amplitude of diurnal cortisol as well.15 Depression symptoms were significantly associated with psychosocial stressors, including greater stress levels about future implications of HD (β = .44, p value = .001) and sleep and psychological difficulties (β = .28, p = .005).16 Presymptomatic HD mutation carriers had a higher salivary morning cortisol level and increased salivary cortisol response compared to symptomatic mutation carriers. There is a correlation between cortisol levels, depression, and symptoms of HD (especially high evening cortisol levels). Moreover, Mo et al. concluded that chronic stress was associated with earlier onset and progression of symptoms of HD in R6/1 transgenic mice.17 Cortisol levels correlate with disease burden score, a predictor of future complications, and the number of years until onset.15
Discussion
In summary, the following environmental factors have demonstrated a correlation between HD diagnosis and the start of symptoms.
Table 1.
Environmental Factor | Correlation to HD Symptoms |
Lifestyle7, 8, 9, 10 | Positive correlation |
Socioeconomic Status11, 12, 13, 14 | Positive correlation |
Mental Health15, 16, 17 | Positive correlation |
Importance of Environmental Correlations with HD
By establishing the association of Huntington’s Disease symptoms with environmental variables, researchers can further investigate the gene-environment interactions that influence the onset of HD symptoms and examine epigenetic interventions to curb the onset or progression of HD symptoms.
Machine Learning
The development of machine learning models may aid data aggregation and analysis by filtering through expansive patient studies while accounting for missing fields and heterogeneous variables. Additionally, these models may offer insight into disease progression patterns overlooked in clinical observation, as Mohan et al. suggest.18
Ganesh et al. analyzed the accuracy of various ML approaches to evaluate HD symptom progression. This study found that the classifier Naive Bayes, which predicts class labels using conditional probabilities, is a prominent choice for effectively discerning gait signals between HD and non-HD individuals, with an accuracy of 94.4% in a diagnostic context. Decision Tree, which uses a decision-based recursive partitioning structure, classified gait signals with an accuracy of 100%. Cross-validated classification accuracy was 90.79% with 4.57% STDEV. Support Vector Machine, which classifies data by finding an optimal boundary between data outputs, differentiated gait patterns among those of different clinical groups (i.e. healthy elderly individuals, post-stroke patients, HD individuals) with an accuracy of 86.9%.19
Furthermore, machine learning can help monitor environmental variables (eg. diet, stress, pollution) that may trigger an earlier onset of HD among HD patients. Cyber-Physical Systems (CPS) can monitor patients’ environments through remote monitoring (eg. through wearable devices) and use genetic testing data to identify individuals at risk of developing HD by considering multiple factors in addition to genetics, offering more specific predictions (eg. age of onset, effective early interventions, and suggested lifestyle modifications).19
Conclusions
By compiling research on environmental variables correlating with HD symptoms, we have demonstrated that HD onset is not solely related to genetics. CAG repeat length has demonstrated a correlation with environmental variables such as stress levels, dietary choices, and other lifestyle decisions. By strengthening existing policies regarding diagnosis and prediction of onset while encouraging further innovation in machine learning, the medical community can strive for more accurate assessments for those affected by HD.
References
- Pringsheim, T., Wiltshire, K., Day, L., Dykeman, J., Steeves, T., & Jette, N. (2012). The incidence and prevalence of Huntington’s disease: A systematic review and meta-analysis. Movement Disorders, 27(9), 1083–1091. https://doi.org/10.1002/mds.25075
- Warby, S. C., Montpetit, A., Hayden, A. R., Carroll, J. B., Butland, S. L., Visscher, H., Collins, J. A., Semaka, A., Hudson, T. J., & Hayden, M. R. (2009). CAG Expansion in the Huntington Disease Gene Is Associated with a Specific and Targetable Predisposing Haplogroup. The American Journal of Human Genetics, 84(3), 351–366. https://doi.org/10.1016/j.ajhg.2009.02.003
- Krobitsch, S., & Kazantsev, A. G. (2011). Huntington’s disease: From molecular basis to therapeutic advances. The International Journal of Biochemistry & Cell Biology, 43(1), 20–24. https://doi.org/10.1016/j.biocel.2010.10.014
- Kremer, B., Goldberg, P., Andrew, S. E., Theilmann, J., Telenius, H., Zeisler, J., Squitieri, F., Lin, B., Bassett, A., Almqvist, E., Bird, T. D., & Hayden, M. R. (1994). A Worldwide Study of the Huntington’s Disease Mutation: The Sensitivity and Specificity of Measuring CAG Repeats. New England Journal of Medicine, 330(20), 1401–1406. https://doi.org/10.1056/nejm199405193302001
- Ferguson, M. W., Kennedy, C. J., Palpagama, T. H., Waldvogel, H. J., Faull, R. L. M., & Kwakowsky, A. (2022). Current and Possible Future Therapeutic Options for Huntington’s Disease. Journal of Central Nervous System Disease, 14(35615642), 117957352210925. https://doi.org/10.1177/11795735221092517
- Lund, C., Breen, A., Flisher, A. J., Kakuma, R., Corrigall, J., Joska, J. A., Swartz, L., & Patel, V. (2010). Poverty and common mental disorders in low and middle income countries: A systematic review. Social Science & Medicine, 71(3), 517–528. https://doi.org/10.1016/j.socscimed.2010.04.027
- Jonson, I., Ougland, R., Klungland, A., & Larsen, E. (2013). Oxidative stress causes DNA triplet expansion in Huntington's disease mouse embryonic stem cells. Stem cell research, 11(3), 1264–1271. https://doi.org/10.1016/j.scr.2013.08.010
- Cubo, E., Rivadeneyra, J., Simón-Vicente, L., Aguado, L., Calvo, S., Saiz-Rodríguez, M., Mariscal, N., Muñoz-Siscart, I., Diaz-Piñeiro, D., Miguel-Pérez, I., Gámez-Leyva, G., García-Bustillo, Á., & Martinez-Descalls, A. (2024). The association between lifestyle factors and mortality in Huntington’s disease. Neurología.
- Mo, C., Hannan, A. J., & Renoir, T. (2015). Environmental factors as modulators of neurodegeneration: insights from gene-environment interactions in Huntington's disease. Neuroscience and biobehavioral reviews, 52, 178–192. https://doi.org/10.1016/j.neubiorev.2015.03.003
- Berggren, K. L., Chen, J., Fox, J., Miller, J., Dodds, L., Dugas, B., Vargas, L., Lothian, A., McAllum, E., Volitakis, I., Roberts, B., Bush, A. I., & Fox, J. H. (2015). Neonatal iron supplementation potentiates oxidative stress, energetic dysfunction and neurodegeneration in the R6/2 mouse model of Huntington's disease. Redox biology, 4, 363–374. https://doi.org/10.1016/j.redox.2015.02.002
- Bruzelius, E., Scarpa, J., Zhao, Y., Basu, S., Faghmous, J. H., & Baum, A. (2019). Huntington’s Disease in the United States: Variation by demographic and socioeconomic factors. Movement Disorders: Official Journal of the Movement Disorder Society, 34(6), 858–865. https://doi.org/10.1002/mds.27653
- Mendizabal, A., Singh, A. P., Perlman, S., Brown, A., & Bordelon, Y. (2023). Disparities in Huntington Disease Severity. Neurology. Clinical Practice, 13(6). https://doi.org/10.1212/cpj.0000000000200200
- Murillas, J. P., Rocha, N. P., Shayan Zadegan, Duncan, B., Ramphul, R., Anjail Sharrief, & Furr-Stimming, E. (2024). The Impact of Social Determinants of Health in the Morbidity of Patients with Huntington Disease (P6-3.001). Neurology, 102(17_supplement_1). https://doi.org/10.1212/wnl.0000000000204947
- The U.S.-Venezuela Collaborative, Wexler, N. S., Lorimer, J., Porter, J., Gomez, F., Moskowitz, C., Shackell, E., Marder, K., Penchaszadeh, G., Roberts, S. A., Gayan, J., Brocklebank, D., Cherny, S. S., Cardon, L. R., Gray, J., Dlouhy, S. R., Wiktorski, S., Hodes, M. E., Conneally, P. M., & Penney, J. B. (2004). Venezuelan kindreds reveal that genetic and environmental factors modulate Huntington’s disease age of onset. Proceedings of the National Academy of Sciences, 101(10), 3498–3503. https://doi.org/10.1073/pnas.0308679101
- Novati, A., Nguyen, H. P., & Schulze-Hentrich, J. (2022). Environmental stimulation in Huntington disease patients and animal models. Neurobiology of Disease, 171, 105725. https://doi.org/10.1016/j.nbd.2022.105725
- Bilal, H., Harding, I. H., & Stout, J. C. (2024). The relationship between disease-specific psychosocial stressors and depressive symptoms in Huntington's disease. Journal of neurology, 271(1), 289–299. https://doi.org/10.1007/s00415-023-11982-x
- Mo, C., Renoir, T., & Hannan, A. J. (2014). Effects of chronic stress on the onset and progression of Huntington's disease in transgenic mice. Neurobiology of disease, 71, 81–94. https://doi.org/10.1016/j.nbd.2014.07.008
- Mohan, A., Sun, Z., Ghosh, S., Li, Y., Sathe, S., Hu, J., & Sampaio, C. (2021). A Machine‐Learning Derived Huntington’s Disease Progression Model: Insights for Clinical Trial Design. Movement Disorders, 37(3), 553–562. https://doi.org/10.1002/mds.28866
- Ganesh, S., Chithambaram, T., Krishnan, N. R., Vincent, D. R., Kaliappan, J., & Srinivasan, K. (2023). Exploring Huntington’s Disease Diagnosis via Artificial Intelligence Models: A Comprehensive Review. Diagnostics, 13(23), 3592. https://doi.org/10.3390/diagnostics13233592
About the Author
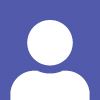
Sophie Ankeles and Amelie Salette are currently juniors at Polytechnic School in Pasadena, CA. Sophie’s interest in genomics started after spending a summer at the Saban Research Institute focusing on neuroepigenetics. In her free time, Sophie enjoys reading and drawing comics. Amelie’s interest in genomics was inspired by her personal experiences with contracting a hereditary disease and her curiosity in examining health policies. She spends her free time crafting pottery pieces and cooking for her family.
Mentor: Dr. Balakrishnan Selvakumar Affiliation: Polytechnic School